Immune Proteins
AlphaFold2, while moving the frontier of protein structure forward, lagged behind predicting antibody structures. As antibodies are a highly desirable drug modality and structures are very important to their in silico engineering and development, acquiring higher quality docking and structure prediction tools remains important. As AlphaFold3 claims superior performance to its predecessor in this domain, Hitawala and Gray, evaluate the improvements, of the new model, along with its shortcomings. AlphaFold3 is by far the best pure structure prediction method available, but there is still significant room for improvement.
The authors find that "AF3 achieves an 8.9% and 13.4% high-accuracy docking success rate for antibodies and nanobodies, respectively, and a median unbound CDR H3 RMSD accuracy of 2.04 Å and 1.14 Å." Ultimately, AF3 fails at 60% of nanobody and antibody docking.
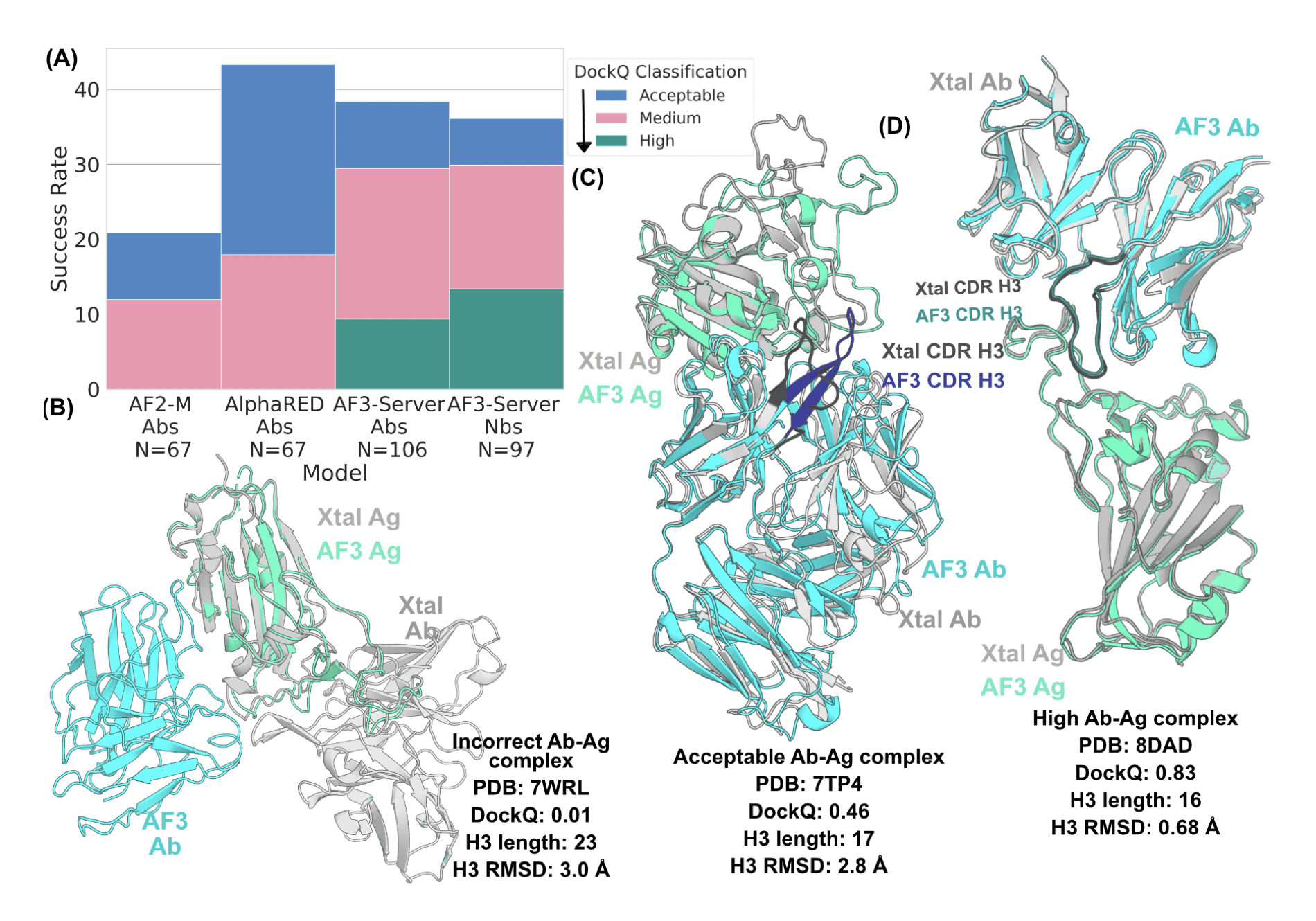
It is that AF3 is considerably better than AF2-Multimer, having significant number of high quality structure predictions observed is 8.9% compared to ~0 for all other methods. While the total number number of "passing" results from AlphaRED exceeds that of AF3, AlphaRED does directly use AF2-Multimer as a starting point. So I remain optimistic that swapping out the AF2-M component of AlphaRED with AF3 might break the 50% success mark.
The authors also demonstrate that the quality of the CDR3 prediction is highly important to the quality of the result, as it is the largest part of the interface between an antibody and antigen. AF3 does show quite strong promise for bound (1.34 Å) and unbound (1.65 Å) CDR3 structures.
Chai-1 Residue Distance Restrictions & Epitope Specification
Chai-1's capabilities extend beyond its base sequence modeling. Users can specify epitopes by identifying maximum distances between two residues in separate chains, for example those retrieved from experimental methods. The authors find that this can improve docking prediction by double digit percentages. Most notably, they find that this epitope limitation doubles antibody-antigen structure prediction accuracy. Try Chai-1 on Tamarind today.
Chai-1r excels in antibody-antigen interaction prediction with mean DockQ % of
- Chai-1r (+4 epitope residues): 43.7
- Chai-1: 35.6
- Boltz (an AlphaFold3 reproduction from MIT & Genesis): 26.4
- AlphaFold2: 20.6

Get In Touch
Tamarind provides a simple, high through graphical interface, and programmatic API. Alongside these, we support chaining dozens of protein design tools. Get in touch to hear more on how we can support your AlphaFold3 usage in a scalable, confidential cloud with enterprise-grade security.